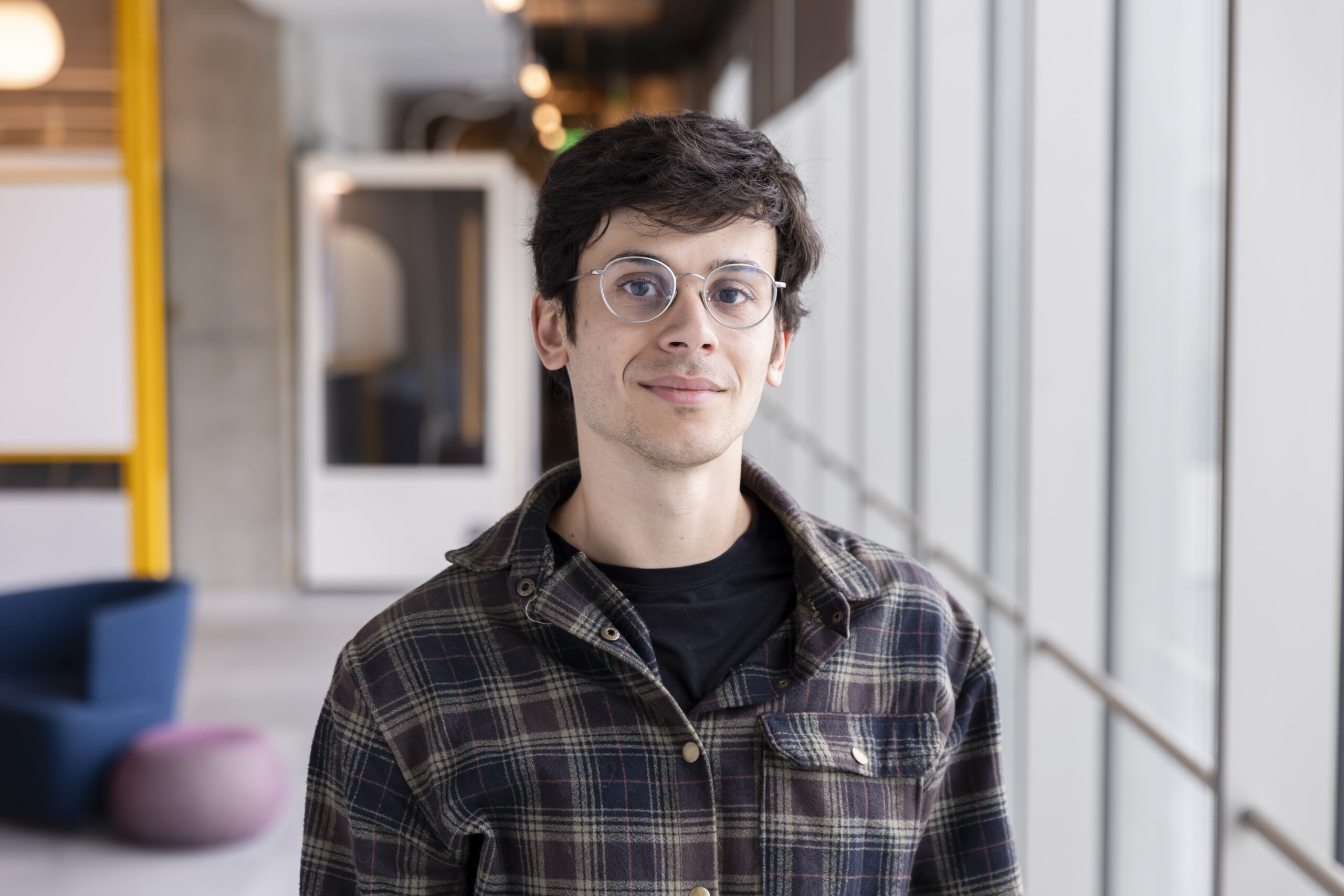
Adam Yala
Electrical Engineering and Computer SciencesAdam Yala is an Assistant Professor in the Department of Electrical Engineering and Computer Sciences.
Project Description
Pillar: Personalized Breast Cancer Screening with Multi-Modal Imaging Risk Models
Joint project with Maggie Chung
National breast cancer guidelines are deemed inefficient, resulting in unnecessary procedures and missed early detection opportunities. Existing risk assessment models, relying on factors such as demographics and family history, often inaccurately predict individual risk. This project proposes a new solution: Pillar, an AI tool that utilizes multi-modal breast imaging data for enhanced risk prediction. Their previous model, Mirai, outperformed existing ones, and by integrating additional imaging data over time, they aim to substantially enhance risk assessment and facilitate early detection and prevention efforts.
Adam Yala’s Story
The Key to Prevention: How a Bakar Fellow Project Aims to Prevent Advanced Breast Cancer Using AI
August 20, 2024
By: Niki Borghei
The AI revolution extends far beyond the flashy headlines of glamorous startups and sophisticated chatbots. In the medical field, for instance, AI is driving significant breakthroughs in healthcare technology. These efforts consist of developing sophisticated tools to improve diagnostics, treatment planning, and patient outcomes. This less visible side of AI is working behind the scenes to enhance the precision and efficiency of medical care, offering transformative potential for addressing some of the most challenging health issues.
Adam Yala is an assistant professor in Computational Precision Health, Statistics, and Computer Science. Along with UCSF radiologist Maggie Chung, they aim to prevent advanced breast cancer through AI-driven personalized screening.
Q: What is the specific need that you are trying to address with your Bakar project?
A: If we can give each patient the right type of screening (e.g. MRI or mammogram) at the right time, we can prevent advanced cancer while reducing the harms of screening (e.g. unneeded biopsies, patient anxiety, and costs). To solve this problem, we need accurate foresight; we need tools to accurately predict patients’ risk of future cancer, and then we need nimble screening guidelines that leverage that information to pre-empt late-stage disease. We also need tools to improve the diagnostic interpretation of those scans and to address the unsustainable shortage of specialized radiologists.
Q: Sounds like a solution is desperately needed! How does yours work?
A: In this project, we’re developing AI tools that can leverage a patient’s prior imaging data, the gigabytes of information in their prior mammograms, MRIs, and tomosynthesis scans, to predict who has cancer today and who will develop cancer in the future. These multi-modal cancer models will support personalized screening guidelines and selective automation of screening exams.
Q: That seems so futuristic! But how exactly are you predicting who will develop cancer?
A: Current guidelines for cancer screening leverage only a few bits of questionnaire-based information (e.g., age, and family history) to determine how a patient should be screened. This results in suboptimal screening recommendations. However, patient care generates gigabytes of data per patient, including longitudinal imaging across multiple modalities (x-ray, MRI, etc.), reflecting a patient’s evolving breast health. Using historical screening data, we’re developing AI tools to predict future cancer from massive longitudinal imaging data.
Q: How did you decide to pursue this area of research?
A: I was inspired by my wonderful PhD advisor, Regina Barzilay. Following her personal experience with breast cancer, Regina was determined to make a difference for future patients. In entering the field, I was shocked at how little we understood of cancer in the clinic; even answering simple questions about how many patients were diagnosed with a particular cancer subtype at a local hospital could take years of work. All that information was buried in clinical notes, and doctors would do months of manual curation to explore research questions. Widely deployed clinical cancer risk models, which underlie national cancer screening recommendations, are terrible at estimating individual cancer risk. While there is a wealth of data in every health system, we were missing the algorithms needed to improve care from it. This sense of “unmet need” is deeply motivating and it drives my research today. During my PhD, I developed Mirai, a mammogram-based risk model, and showed that the model outperformed the clinical state-of- the-art in a global validation study across five countries. Since then Mirai has been validated at 43 hospitals from 14 countries. However, we’re just scratching the surface of what’s possible with AI-driven risk prediction, and bringing the ongoing advances to patients is at the core of our Bakar Spark project.
Q: It’s really inspiring that your advisor sparked this interest in you that will help save so many lives. Now, how did you decide that entrepreneurship would be the way in which you make your impact?
A: Our motivation has always been to change the practice of cancer screening and to improve the lives at scale. This just isn’t something we can do from academia alone; entrepreneurship is the best mechanism for translating new technologies for large-scale and sustainable impact.
Q: How will the Bakar Fellows Program support you in making this a reality?
A: Our Bakar Spark Award will support our brilliant innovation fellows, Krishna Agrawal and Tony Lian, in building our multi-modal cancer risk models. It will also support some of our compute costs in training these large-scale models.
Q: How did this come about as a joint project?
A: I’ve worked with Maggie since first arriving at UC Berkeley/UCSF, and I couldn’t hope for a better partner in this work. As a practicing breast imaging radiologist and a physician-scientist, her clinical expertise is essential in conducting and translating this research. We’ve collaborated on fundraising, forging data partnerships, building models, and running clinical trials. Our collaboration is stellar, and I’m thrilled to continue building together in our Bakar Spark project.
Q: So what’s next?
A: We hope to start disclosing the first inventions as soon as a year from now. We plan to start the commercialization process when the first models are ready. While regulatory timelines are always uncertain, we hope to have our tools on the market within the next few years.