Bakar Fellows Program Announces 2024 Spark Award Recipients and New Partnership with the Academic Innovation Catalyst
By: Niki Borghei •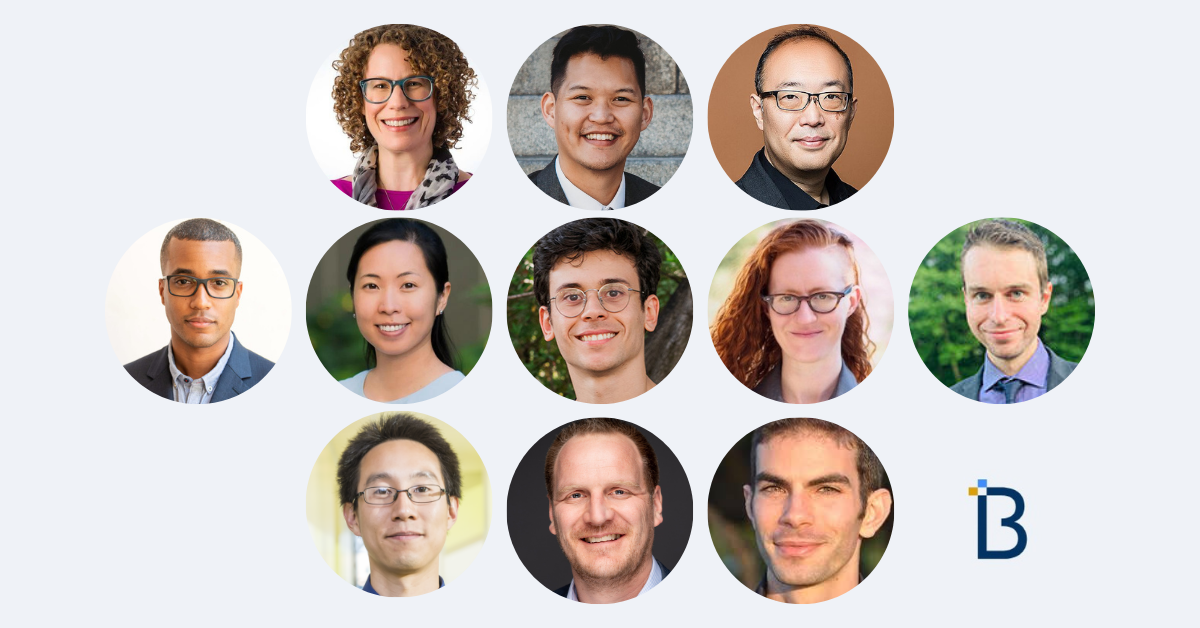
This year’s Spark Award recipients embody the spirit of innovation and collaboration that defines UC Berkeley’s entrepreneurial ecosystem. From therapeutics for cancer patients to more sustainable power sources for the AI revolution, our new cohort of Faculty Fellows have proven their readiness to address society’s greatest challenges.
The Bakar Fellows Program has been committed to expanding access to entrepreneurship since its inception in 2012, providing funding, resources, and mentorship to faculty, graduate students, and, most recently, undergraduates. Last year, the Bakar Fellows Program was pleased to announce a new component of the program that encourages partnerships between UC Berkeley and UC San Francisco faculty, facilitating the transformation of cutting-edge health research into market-ready solutions.
This year marks the beginning of a new partnership with the Academic Innovation Catalyst (AIC), a new deep tech funding platform founded by Matt and Lisa Sonsini. Initially, AIC will join forces with the Bakar Fellows program to allocate grants to Berkeley faculty immersed in deep technology exploration, particularly in climate technology. Grant recipients will receive $300,000 over three years for market research, prototype development and other commercialization activities, in line with the standard Bakar Fellows award amount and duration. AIC envisions expanding this pilot initiative across UC Berkeley and beyond, fostering innovation on a broader scale within the academic community.
The inaugural recipients of the AIC grants are Robert Pilawa-Podgurski, a UC Berkeley Electrical Engineering & Computer Sciences professor, and Zakaria Al Balushi, a UC Berkeley Materials Science and Engineering assistant professor.
Learn more about the 2024 Spark Award recipients and their projects below:
- Zakaria Al Balushi, Rare-Earth Catalysts for Low Temperature Production of Nitrogen Enriched Materials and Chemicals of High Value
Producing nitrogen-rich materials and chemicals typically requires significant energy. This project introduces a greener and more cost-effective option for producing these high-value materials and chemicals, including gallium nitride, a material used in semiconductors, and ammonia, which currently requires 2% of the world’s energy usage for production.
- Liana Lareau, Engineering Poison Exons to Treat Repeat Expansion Diseases
This project focuses on developing kill switches to deactivate disease-causing genes, particularly focusing on challenging conditions like Huntington’s disease. Standard CRISPR approaches for these conditions have limitations. Instead, the project proposes a novel method utilizing machine learning and CRISPR editing to insert ‘poison exons’ into disease genes. These exons, when incorporated into mRNA, eliminate toxic proteins responsible for the disease. This approach offers a versatile platform for targeting various genetic diseases, particularly repeat expansion disorders.
- Jennifer Listgarten (UCB) and Andrew Yang (UCSF) , Revealing the Molecular Code for Crossing the Blood-Brain Barrier
New studies have demonstrated that the blood brain barrier, known for protecting the brain from the bloodstream, is not as impermeable as once thought. For example, brain function is remarkably sensitive to the signals in the blood, and there are proteins, such as transferrin, that are able to permeate the blood brain barrier. This project aims to further investigate brain-permeable proteins and receptors in the blood brain barrier with the goal of utilizing built-in transport pathways to deliver therapies for treating all kinds of neurological disease.
- Daniel Nomura, Drugging Oncogenic Transcription Factors
This proposal aims to address the challenge of targeting transcription factors (TFs), which are crucial in cancer but difficult to drug due to their unique structure. The proposed solution involves using new methods to develop drugs that can target these TFs effectively. These methods include techniques like using covalent chemoproteomics, molecular glue degraders, and targeting TFs with specific proteins in the cell. This approach aims to produce better treatments for cancer patients and possibly others who need therapies targeting these hard-to-reach TFs.
- Robert Pilawa-Podgurski, Powering the AI Revolution: A New Voltage Regulator Enabling Direct 48-V-to-1-V Vertical Power Delivery for Next-Generation Ultra-High-Power Processors
High-performance processors are crucial for technologies like AI and autonomous vehicles, but their power usage is soaring, approaching 1000 W. This proposal seeks to make use of innovative tools and techniques designed in Pilawa-Podgurski’s lab to develop a hybrid switched-capacitor voltage regulation module for ultra-high-power processors that can achieve a 2x reduction in power conversion losses and a 4x reduction in physical size compared to state-of-the-art commercial products. This could reduce overall data center power usage by almost ten percent, benefiting both the environment and businesses.
- Robert Saxton, Leveraging AI-Based De Novo Protein Design to Overcome Leptin-Resistant Obesity
Obesity impacts over 40% of adults and 20% of children in the US and costs the healthcare system billions of dollars annually. While recent GLP-1-based therapies have shown impressive results in promoting weight loss, concerns around side-effects and other limitations suggest that new modalities are still needed. Leptin, a hormone regulating body weight and metabolism, offers potential, but leptin resistance complicates its effectiveness. This proposal aims to use AI-guided protein design to create targeted leptin receptor biased agonists, in order to overcome leptin resistance and promote safe and sustained weight loss and metabolic health.
- Joseph Shapiro, Machine Learning Simplifies Compliance with Government Regulation
This project aims to reduce costs and uncertainty for firms complying with government regulations, initially focusing on the US Clean Water Act. This law regulates “Waters of the United States,” but lacks clarity on specific covered areas. As a result, developers often face costly delays and compliance expenses. The solution is a machine learning algorithm predicting the likelihood of Clean Water Act regulation for any US location. It utilizes ground-truth data from the Army Corps and various geophysical layers, achieving high accuracy.
- Patrick Shih, Transforming the Paradigm of Fungal Biomanufacturing
This project proposes a platform which addresses the challenge of genetically modifying non-model and lesser-studied fungal species, important for making a range of compounds in industries like pharmaceuticals, biofuels, and agriculture. Currently, these fungi lack the tools needed for efficient manipulation, which limits their commercial potential. By improving their genetic flexibility, they enable the use of their unique abilities, making it easier to produce different compounds for various purposes in human health, agriculture, and bioenergy.
- Adam Yala (UCB) and Maggie Chung (UCSF), Pillar – Personalized Breast Cancer Screening with Multi-Modal Imaging Risk Models
National breast cancer guidelines are deemed inefficient, resulting in unnecessary procedures and missed early detection opportunities. Existing risk assessment models, relying on factors such as demographics and family history, often inaccurately predict individual risk. This project proposes a new solution: Pillar, an AI tool that utilizes multi-modal breast imaging data for enhanced risk prediction. Their previous model, Mirai, outperformed existing ones, and by integrating additional imaging data over time, they aim to substantially enhance risk assessment and facilitate early detection and prevention efforts.
Click here to access the Academic Innovation Catalyst press release.